有关叶片排序问题的研究
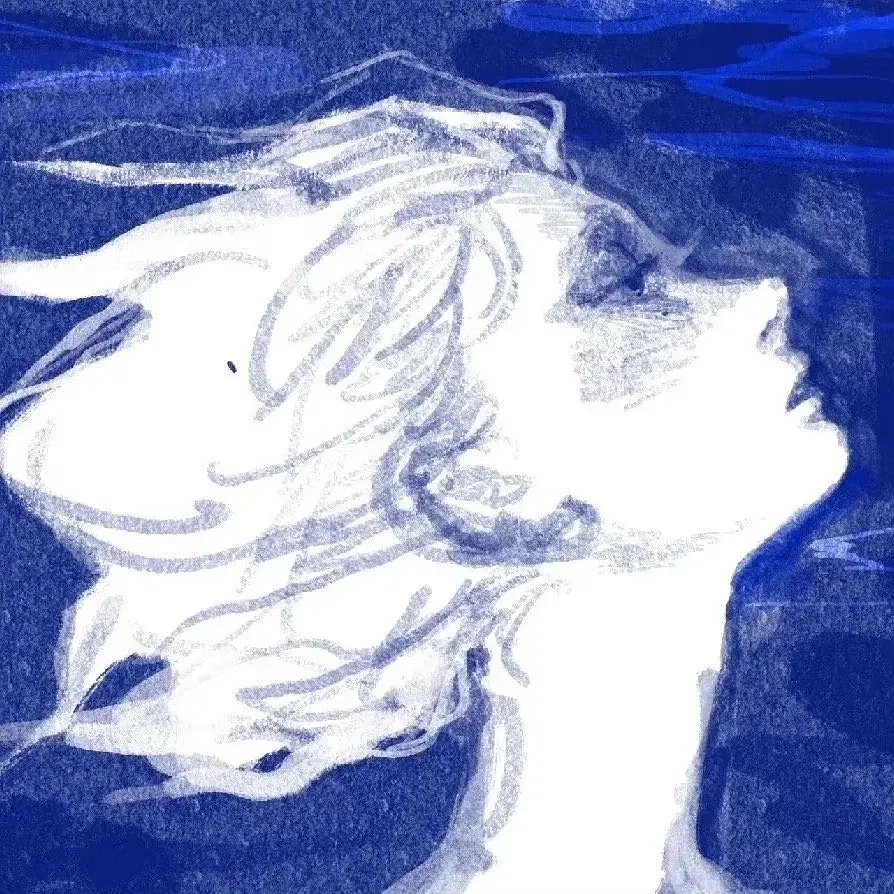
文献综述:
叶片通常数量十分多,所有排列的组合有
李宏亮等人采用遗传算法研究汽轮机转子多级叶片不平衡优化问题^1,通过优化,使得水平力矩降至优化前的
同时许多启发式算法有很多的创新,如Zhang et al.提出了一种基于强化学习的自适应优化算法,能在动态变化的优化问题中保持良好的表现,适用于求解非线性、非凸问题[^4]。强化学习在求解复杂优化问题中逐渐显示出强大的潜力,尤其是在多目标优化任务中。Tarek et al.研究了粒子群优化(PSO)算法在复杂组合优化问题中的应用,并提出了一种自适应参数调整策略,显著提高了算法的收敛速度和解的精度[^5]。PSO作为一种全局优化方法,其在处理连续和离散优化问题时都展现了较强的能力。Xie et al.提出了一种混合智能算法,结合遗传算法和模拟退火算法(GA-SA),该方法能够在复杂环境下平衡探索与开发,提高了解的稳定性和质量[^6]。该方法被应用于结构优化问题,结果表明其优越性。
为了克服全局搜索算法的低效,一种局部搜索算法应运而生。Brito等人提出了迭代局部搜索(ILS)算法[^7],该方法在每次陷入局部最优解时,从当前解的邻域随机选择一个解进行转移,并通过多次执行扰动步骤,跳出局部最优,提升了局部搜索的能力。刘义等人在ILS的基础上提出了TILS算法[^8],采用了阈值限定的扰动策略,将随机扰动和局部最优的跳出机制结合起来,进一步提高了搜索效率和解的质量。Pullan等人提出的动态局部搜索算法[^9],通过引入惩罚函数,能够在局部最优附近进行有效探索,避免陷入局部极小值。
尽管上述启发式算法取得了显著的进展,但它们依然是基于近似求解,因此在求解精确解方面存在一定的局限性。为此,如何设计一种能够更精确求解的算法,仍然是一个值得深入研究的问题。
[^2]: 赵天宇, 袁惠群, 杨文军, 张宏远. 非线性摩擦失谐叶片排序并行退火算法[J]. 航空动力学报, 2016, 31(5): 1053-1064. doi: 10.13224/j.cnki.jasp.2016.05.005
[^4]: Zhang, Y., Liu, J., & Wang, Z. (2021). Adaptive optimization algorithm based on reinforcement learning for nonlinear problems. Journal of Computational Physics, 434, 109310. DOI:10.1016/j.jcp.2021.109310
[^5]: Tarek, M., & Zhao, J. (2019). Particle swarm optimization with adaptive parameters for complex optimization problems. Applied Soft Computing, 80, 561-570. DOI: 10.1016/j.asoc.2019.04.042
[^6]: Xie, H., Li, Q., & Li, Y. (2020). Hybrid GA-SA algorithm for complex structural optimization. Structural and Multidisciplinary Optimization, 61(5), 1247-1258. DOI: 10.1007/s00158-019-02481-w
[^7]: BRITO J, OCHI L, MONTENEGRO F, et al. An iterative local search approach applied to the optimal stratification problem[J]. International Transactions in Operational Research,2010,17(6): 753-764.
[^8]: 刘谊, 郭闯强, 朱映远, 等. 基于 TILS 算法的汽轮机叶片排序方法[J]. 航空动力学报, 2024, 39(X):20220612.LIU Yi, GUOChuangqiang, ZHU Yingyuan, et al. Steam turbine blade sequencing method based on TILS algorithm[J]. Journal of Aerospace Power,2024, 39(X):20220612.
[^9]: PULLAN W, HOOS H H. Dynamic local search for the maximum clique problem[J].Journal of Artificial Intelligence Research, 2006, 25: 159-185
背景
选题依据:
在工程设计中,特别是涉及旋转部件如涡轮机或风扇时,叶片的排列对设备的平衡和性能至关重要。不平衡的叶片排列可能导致振动、噪音增加以及部件寿命缩短。因此,优化叶片排列以最小化质量矩,从而实现最佳平衡,是提高设备效率和可靠性的关键。
研究方案:
- 内容:学习遗传算法和拉格朗日对偶算法,结合问题模型,求(近似)最优排序方法
- 目标:寻求叶片排序问题的最优解
- 拟采用方法: 遗传算法 拉格朗日对偶
- 关键问题:拉格朗日对偶如何使用;遗传算法如何优化
预期实现目标:
优化遗传算法,使结果无限趋近最优解
成功建立拉格朗日对偶算法的模型,并求解答案
研究实施计划:
- 理解问题,初步建立数学模型 ——2周
- 学习相关算法,并编写算法程序供求解使用 ——6周
- 将数据带入程序求解 ——1周
- 分析解得数据,对结果进行检验 ——1周
研究过程
问题建模
决策变量 :
用二元变量 (
目标函数 :
目标是最小化总质量矩:
约束条件 :
每个叶片必须在一个位置上:
每个位置上只能有一个叶片:
质量m/g:
半径:
算法学习
遗传算法学习
遗传算法(Genetic Algorithm, GA)是一种模拟自然选择和遗传机制的搜索启发式算法。它通过模拟生物进化过程中的选择、交叉和变异等操作,对候选解进行迭代优化,以期找到问题的最优或近似最优解。遗传算法具有较强的全局搜索能力,能够在大规模搜索空间中高效地寻找近似最优解,比较适合解决该研究问题。
我基于一个简单案例 展开学习 如下:
1 | ## 案例描述 |
基于这个简单的案例,我测试了更复杂的Sphere函数和Rosenbrock函数,搜索空间设置在[-10,10].
函数定义如下
Sphere 函数
Sphere 函数定义为:
该函数的最小值为 0,发生在
Rosenbrock 函数
Rosenbrock 函数定义为:
该函数的最小值为 0,发生在
具体修改步骤:
部分函数的修改:将适应度函数作为参数传递给
GeneticAlgorithm
类,在初始化时设置fitness_function
。1
2
3
4
5
6
7
8
9
10
11
12
13
14
15
16
17
18
19
20
21
22
23
24
25
26
27
28
29
30
31
32
33
34
35
36
37
38
39
40
41
42
43
44
45
46
47
48
49
50
51
52
53
54
55
56
57
58
59
60
61import random
import numpy as np
class GeneticAlgorithm:
def __init__(self, population_size, generations, mutation_rate, crossover_rate, gene_length, bounds, fitness_function):
self.population_size = population_size
self.generations = generations
self.mutation_rate = mutation_rate
self.crossover_rate = crossover_rate
self.gene_length = gene_length
self.bounds = bounds
self.fitness_function = fitness_function
self.population = self._initialize_population()
def _initialize_population(self):
population = []
for _ in range(self.population_size):
individual = [random.uniform(self.bounds[i][0], self.bounds[i][1]) for i in range(self.gene_length)]
population.append(individual)
return population
def fitness(self, individual):
return self.fitness_function(individual)
def selection(self):
total_fitness = sum(self.fitness(ind) for ind in self.population)
probabilities = [self.fitness(ind) / total_fitness for ind in self.population]
selected_parents = np.random.choice(self.population, 2, p=probabilities)
return selected_parents
def crossover(self, parent1, parent2):
if random.random() < self.crossover_rate:
point = random.randint(1, self.gene_length - 1)
child1 = parent1[:point] + parent2[point:]
child2 = parent2[:point] + parent1[point:]
return [child1, child2]
else:
return [parent1, parent2]
def mutate(self, individual):
if random.random() < self.mutation_rate:
index = random.randint(0, self.gene_length - 1)
individual[index] = random.uniform(self.bounds[index][0], self.bounds[index][1])
return individual
def evolve(self):
for generation in range(self.generations):
new_population = []
for _ in range(self.population_size // 2):
parents = self.selection()
offspring = self.crossover(parents[0], parents[1])
new_population.extend([self.mutate(child) for child in offspring])
self.population = new_population
best_individual = min(self.population, key=lambda ind: self.fitness(ind))
print(f"Generation {generation}: Best Fitness = {self.fitness(best_individual)}")
best_individual = min(self.population, key=lambda ind: self.fitness(ind))
return best_individual修改适应度函数:将适应度函数分别按照函数表达式进行修改,代码如下:
1
2
3
4
5
6
7
8# 定义 Sphere 函数的适应度
def sphere_fitness(individual):
return sum(x ** 2 for x in individual)
# 定义 Rosenbrock 函数的适应度
def rosenbrock_fitness(individual):
x1, x2 = individual
return (1 - x1) ** 2 + 100 * (x2 - x1 ** 2) ** 2搜索空间:
bounds=[(-10, 10), (-10, 10)]
表示每个变量或 的值在区间 [−10,10] 内。 运行测试:
首先运行 Sphere 函数的测试,采用三维空间。
然后运行 Rosenbrock 函数的测试,采用二维空间。
主程序如下:
1
2
3
4
5
6
7
8
9
10
11
12
13
14if __name__ == "__main__":
# 测试 Sphere 函数
ga_sphere = GeneticAlgorithm(population_size=30, generations=200, mutation_rate=0.05,
crossover_rate=0.7, gene_length=3, bounds=[(-10, 10), (-10, 10), (-10, 10)],
fitness_function=sphere_fitness)
best_solution_sphere = ga_sphere.evolve()
print(f"Best Solution for Sphere: {best_solution_sphere}")
# 测试 Rosenbrock 函数
ga_rosenbrock = GeneticAlgorithm(population_size=30, generations=200, mutation_rate=0.05,
crossover_rate=0.7, gene_length=2, bounds=[(-10, 10), (-10, 10)],
fitness_function=rosenbrock_fitness)
best_solution_rosenbrock = ga_rosenbrock.evolve()
print(f"Best Solution for Rosenbrock: {best_solution_rosenbrock}")
运行结果:
对于 Sphere 函数,最优解 (0,0,0),最小值为 0。
于 Rosenbrock 函数,最优解 (1,1),最小值为 0。
拉格朗日对偶算法学习
基本思想:
1. 将约束约束放到目标上去
2. 尽量松弛掉耦合约束(linked/coupling constraints) 让不可分问题变为可分问题
松弛后的子问题求解:
暴力求解/求解器/动态规划/线性规划
拉格朗日乘子的更新 (对偶问题求解):
由于对偶问题是一个非光滑凸优化问题,采用次梯度算法来更新拉格朗日乘子。
学习实例 :
简单二次整数规划问题:
将两个约束松弛到目标函数上来可得
$$
L(x_1,x_2,x_3,x_4,x_5,x_6,λ_1,λ_2) \
=0.5x_1^2+0.1x_2^2+0.5x_3^2+0.1x_4^2+0.5x_5^2+0.1x_6^2\
+λ_1(48−x_1+0.2x_2−x_3+0.2x_4−x_5+0.2x_6)\
+λ_2(250−5x_1+x_2−5x_3+x_4−5x_5+x_6)
$$
代码主流程如下(具体代码见链接):
1 | max_itertimes = 30 |
问题求解
遗传算法
步骤
初始化种群
首先需要生成一个初始种群,种群中的每个个体代表一个可能的叶片排列顺序。
1 | # 初始化种群 |
适应度函数
适应度函数用于评估个体的优劣。对于本问题,适应度函数则是计算给定排列的质量矩大小,质量矩越小,适应度越高。
给定排列的质量矩大小的计算:通过将每个叶片的质量与其在圆周上的位置角度相结合,分别计算质量在x和y方向上的投影,然后求平方和的平方根。
1 | # 适应度函数:计算给定排列的质量矩 |
锦标赛选择
选择操作从当前种群中选择个体进入下一代。锦标赛选择是一种常用的选择机制,每次从种群中取出一定数量个体(放回抽样),然后选择其中最好的一个进入子代种群。重复该操作,直到新的种群规模达到原来的种群规模。
1 | # 选择(锦标赛选择)随机选择一定数量的个体(通常称为“锦标赛”),然后从中选择适应度最好的个体作为下一代的父母 |
交叉方法
交叉操作用于生成新的个体。
基因重组后产生的后代可能出现编码重复的情况,那么就需要我们对产生的子代进行修订这里我们使用部分匹配交叉(PMX)
PMX是一种适用于排列问题的交叉操作,能够保持父代个体的部分基因顺序。
实现逻辑 如下:
1:随机选择一对染色体(父代)中几个基因的起止位置(两染色体被选位置相同)
2:交换这两组基因的位置。
3:做冲突检测,根据交换的两组基因建立一个映射关系。最后所有冲突的基因都会经过映射,保证形成的新一对子代基因无冲突。
1 | # 交叉(部分映射交叉 PMX)目标是在两个父代个体之间交换部分基因片段,同时保持基因片段外的其他位置的相对顺序不变。 |
变异操作(交换变异)
变异操作可以引入随机变化,从而增加种群的多样性。
假设有一个个体基因发生变异,那么基因肯定会出现重复。为了修正重复,故将重复的基因修改为原变异基因变异前的基因。
即变异操作通过随机交换个体中的两个基因来实现。
实际意义为任意选择两个叶片交换位置。
1 | # 变异(交换变异) |
遗传算法主流程
遗传算法的主要流程包括初始化种群、评估适应度、选择、交叉、变异等步骤。
同时,也会在每一次迭代时更新以及记录最优解以及每200代输出一次结果,以便于观测。
将这些这些步骤重复进行,直到达到设定的迭代次数。
1 | # 遗传算法主流程 |
可视化
1 | # 绘制质量矩随迭代的变化 |
设定
基本库导入
1 | import numpy as np |
参数
1 | # 参数 |
结果分析
可以通过修改参数,寻找合适的参数以获得更优结果,相关测试如下表:
组数 | pop_size | num_generations | mutation_rate | 最小质量矩 未乘半径 |
---|---|---|---|---|
1 | 200 | 5000 | 0.5 | 0.000379928 |
2 | 300 | 6000 | 0.5 | 0.000106675 |
3 | 500 | 5000 | 0.5 | 0.000209263 |
4 | 500 | 5000 | 0.6 | 0.000070014 |
选取第四组数据,进行20次测试,结果如下:
最大值 | 最小值 | 平均值 | 方差开根 |
---|---|---|---|
0.000230939 | 0.000070014 | 0.0001508253 |
对应的图像
![]() |
![]() |
![]() |
![]() |
分析
由结果可见当种群大小较大,变异率在0.6附近时可以获得
同时结合运算20次的结果可知该代码效果较稳定。
而且由图像可知,收敛速度十分快。在前100代就实现缩小到最初质量矩的20%~25%
结果
最优排列: [(17.23), (17.39), (17.51), (17.49), (17.35), (17.32), (17.34), (17.47), (17.37),
(17.31), (17.86), (17.58), (17.28), (17.27), (17.29), (17.54), (17.15), (17.45),
(17.52), (17.43), (17.41), (17.38), (17.42), (17.56), (17.48), (17.46), (17.36)]
未来
可以修改变异的逻辑,比如每次交换对称位置的叶片。
进一步优化参数,以获得更精确的结果
可以考虑将算法扩展到更复杂的情景,如多目标优化或考虑更多约束条件。
拉格朗日松弛算法
遇到的困难&解决方案
在研究过程中 我发现 对于这个问题 很难使用纯粹的拉格朗日松弛算法,
首先,我们有目标函数:
为了简化问题,我们可以考虑最小化 (
接下来,针对两个约束,我们可以构造拉格朗日函数:
为了找到极小值,我们需要对每个 (
首先,计算 (
因此:
整理一下:
这个方程对于每个 ( i ) 和 ( j ) 都要成立。然而,实际的优化问题是一个整数线性规划问题,其中的
同时,我们选择松弛分配约束,导致构造的拉格朗日函数十分复杂,求解这个子问题同样十分困难。
综上所述,拉格朗日松弛算法很难直接利用。
于是我保留了部分拉格朗日松弛算法的思想,将构造的拉格朗日函数添加项视为罚函数,然后结合局部搜索算法求解。
其中局部算法产生是因为在求解过程中,我首先注意到每一代运算结果不一定是优化,可能会出现负优化的情况。于是我添加了记录最优解,代代更新的策略。继续求解的过程中我发现当代数超过一定数目后,结果不会改变,即陷入局部最优解情况。于是我添加了停滞检测程序,当结果持续不变且未达到设定精度时,会对排序进行一次部分扰动,以探索更多解空间。然后我发现当设定的精度过小时,哪怕迭代很多代也无法实现,因此我设定了一个最大运行代数,以避免死循环。
在后续学习过程中,我发现这个思想与局部搜索算法十分相像。
核心代码如下 包含具体注释:
1 | # 定义主函数lagrangian_relaxation |
结果分析
当tolerance=0.0002
时,普遍可以在20000次左右得到结果。
该算法运行速度十分快,迭代10万次6 s左右,相较于遗传算法在获得相同精度时需要2 min左右,速度快了100多倍。
因此可以进一步提高精度,
当tolerance=0.0001
时,不到一分钟便可以获得0.00004846087的高精度结果。
进一步测试算法极限发现,该算法设定最大代数为100万次,可获得最好结果在0.00005附近。
运行时间在1分钟左右。
可以通过调大最大迭代次数以获得更精确的结果。
![]() |
![]() |
结果
未来
结合局部搜索算法的引入,该算法有许多优化之处:
禁忌搜索:维护一个禁忌表,记录最近访问过的解,避免重复访问,促进更广泛的探索。
设置多重起始点:从多个随机初始排列开始优化,选择其中质量矩最小的排列作为最终解。
并行计算:利用多线程或分布式计算来同时评估多个排列,加速搜索过程。
指针网络(仅学习)
指针网络(Pointer Networks,简称Ptr-Nets)是一种特殊的神经网络架构,它被设计用于处理输入序列和输出序列之间存在直接对应关系的问题。传统的序列到序列模型(Seq2Seq)通常将输出限制为一个固定的词汇表中的词,而指针网络则可以将输出的每个元素指向输入序列中的某个元素,这使得它们非常适合于那些需要从输入中选择元素作为输出的任务。
指针网络特别适用于以下几种情况:
可变大小的输出:当输出序列的长度取决于输入序列时,使用固定大小的词汇表的传统模型会遇到困难。而指针网络能够灵活应对这种变化。
稀疏输出:当输出是输入的一个子集或排列时,传统模型可能会因为输出空间过大而难以训练。指针网络通过直接在输入序列上操作,避免了这个问题。
组合优化问题:例如旅行商问题(TSP),其中任务是找到访问所有给定点并返回起点的最短路径。这类问题通常具有离散且结构化的解空间,指针网络可以通过学习如何选择下一个访问点来解决这些问题。
工作原理
编码器(Encoder):类似于标准的序列到序列模型,指针网络首先使用RNN(如LSTM或GRU)对输入序列进行编码,产生一系列隐藏状态。
解码器(Decoder):解码器也基于RNN,并在每一步生成一个注意力分布(attention distribution)。这个分布不是用来预测下一个单词的概率,而是用作指针,指向输入序列中的一个特定位置。因此,解码器的输出是一个索引,指示了输入序列中的哪个元素应该被选中。
注意力机制(Attention Mechanism):这是指针网络的核心部分。它计算当前解码步骤与所有编码隐藏状态之间的相似度得分,然后应用softmax函数将这些得分转换成概率分布。最终,该分布用于确定哪个输入元素应当被“指向”。
训练指针网络
在训练过程中,指针网络通常采用监督学习的方式,即提供带有正确答案的数据对(输入序列及其对应的输出序列)进行训练。损失函数一般选择交叉熵损失,以衡量预测的指针位置与真实位置之间的差异。
指针网络已经被证明在多种任务上有效,包括文本摘要、问答系统以及各种组合优化问题。如果你正在寻找一种方法来处理涉及从输入序列中选择或排列元素的任务,那么指针网络可能是一个值得考虑的选择。
环境配置
虚拟环境:miniconda
python版本 :不高于3.7
tensor flow版本 :1.13.1 pip install tensorflow==1.13.1
插件:
- 数据处理:
numpy
- 数据可视化:
matplotlib
- 进度条工具:
tqdm
结果分析

不过该算法运行速度十分慢,原因之一是下载的tensor flow为CPU版本,可以配置GPU版本优化。
总结
综上三种算法各有优劣,其中效果较好且运行相对较快的算法是拉格朗日松弛算法。在未来可以进一步通过优化参数,或者结合其他优化算法来实现更高效的求解。
- Title: 有关叶片排序问题的研究
- Author: metriver
- Created at : 2024-11-30 00:00:00
- Updated at : 2024-12-30 19:18:29
- Link: https://redefine.ohevan.com/2024/11/30/科研课堂 研究报告/
- License: This work is licensed under CC BY-NC-SA 4.0.